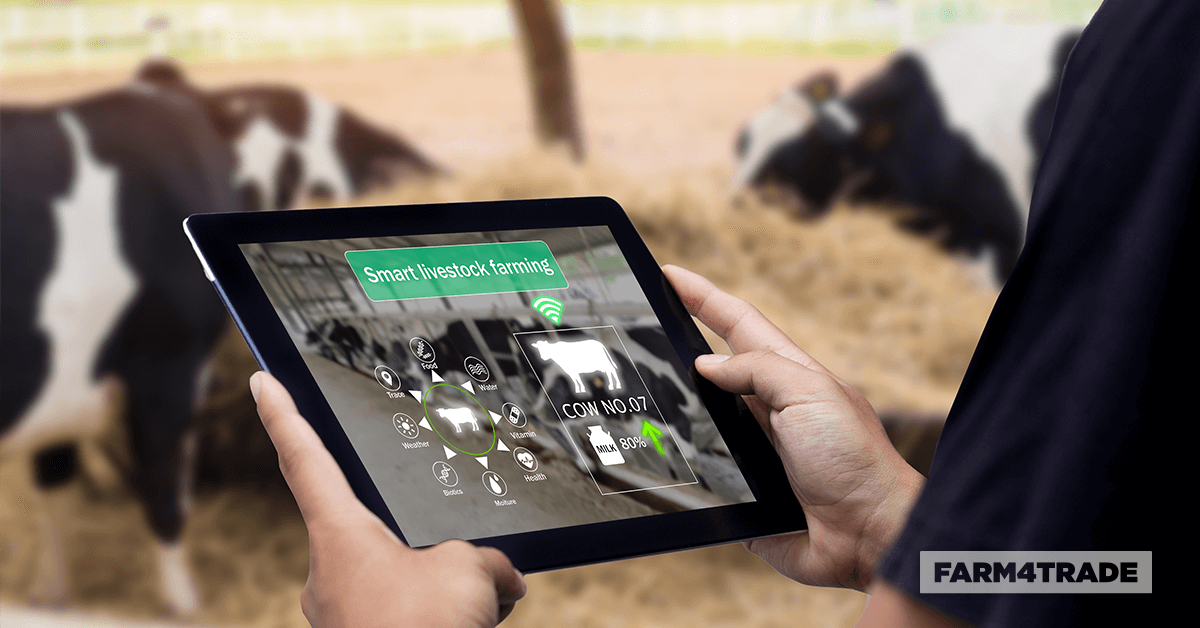
Farm4Trade has chosen, since its founding in 2016, to invest in R&D, research and technology development, as a priority over any other purpose, aiming at creating accessible innovations based on first class coding models.
Part of Farm4Trade’s team is actually based at the University of Modena and Reggio Emilia and is completely dedicated to research and development activities on artificial intelligence, machine learning and data modeling within the AlmageLab, Department of Engineering “Enzo Ferrari”, that is labelled as an international pole of excellence in computer vision and artificial intelligence.
Academic research has led so far to great leaps for two of our R&D projects.
Our ADAL technology (Automatic Detection of Abattoir Lesions) is a breakthrough Artificial Intelligence system capable of automating a photo acquisition process on half-carcasses at the abattoir, while classifying lesions present on the organs of the slaughtered animals. This project is the first animal production system to apply deep learning techniques with the scope of significantly elevating environmental friendly and sustainable food production. As a first step, ADAL’s goal is to develop and deploy an automated mass data capture system for respiratory diseases in slaughtered pigs. The first prototype is expected to identify 95% of diseased carcasses.
ADAL is currently demonstrated ready to be used in an operational environment.
Our PhAID technology is a contactless biometric (photographic) recognition system for the identification of animals, as a technology to be integrated in all Farm4Trade tools and services, already completed or to be developed. This new approach will facilitate livestock traceability, following the animal's health from the birth until the slaughter, resulting in a suited monitoring and control tool able to fill both farmers and stakeholders’ needs.
Phaid has currently system completed and qualified, reliability and optimization tests in real operative environment are underway.
Nowadays, most research on identification methods via artificial intelligence is focused on people rather than animals, mainly due to the development of better images features extraction using deep convolutional neural networks and the availability of large datasets employed to train such networks.
Our research activities instead focus on the use of deep learning techniques, and particularly to the domain adaptation techniques, in contactless biometric scans. We study the methods of adaptation for a dataset of limited size and the methods of adapting pre-trained models in different domains, in depth .
We are confident in saying that the more complex and sophisticated the systems for animal identification get, the more they can be reversed and applied to the human field.
That is the case of the recent publication carried on by three members of our research team, Luca Bergamini, Angelo Porrello and Simone Calderara.
They contributed to the 2020 European Conference on Computer Vision (ECCV 2020), the top european conference in the image analysis area, with a paper on re-identification obtained by a groundbreaking application of the teacher-scholar framework to multiple views, rather than temporal shifted frames of the same view, using an experimental technique called Views Knowledge Distillation (VKD) that is able to perform robust re-identification on smaller dataset of images.
This method has been applied to various domains, in spite of single domains predominant Computer Vision applications, and instead achieves strong results on multiple domains, allowing Person, Vehicle and Animal re-identification.
Expanding the field of research and application of these findings, which are already embedded in our technologies in a real life functional context as described above, we are certainly aware of how disruptive and ground breaking they may sound now, but they will be soon adopted as the gold standard for identification and re-identification and embedded in industrial utilization.